What Is Analysis of Variance (ANOVA)?
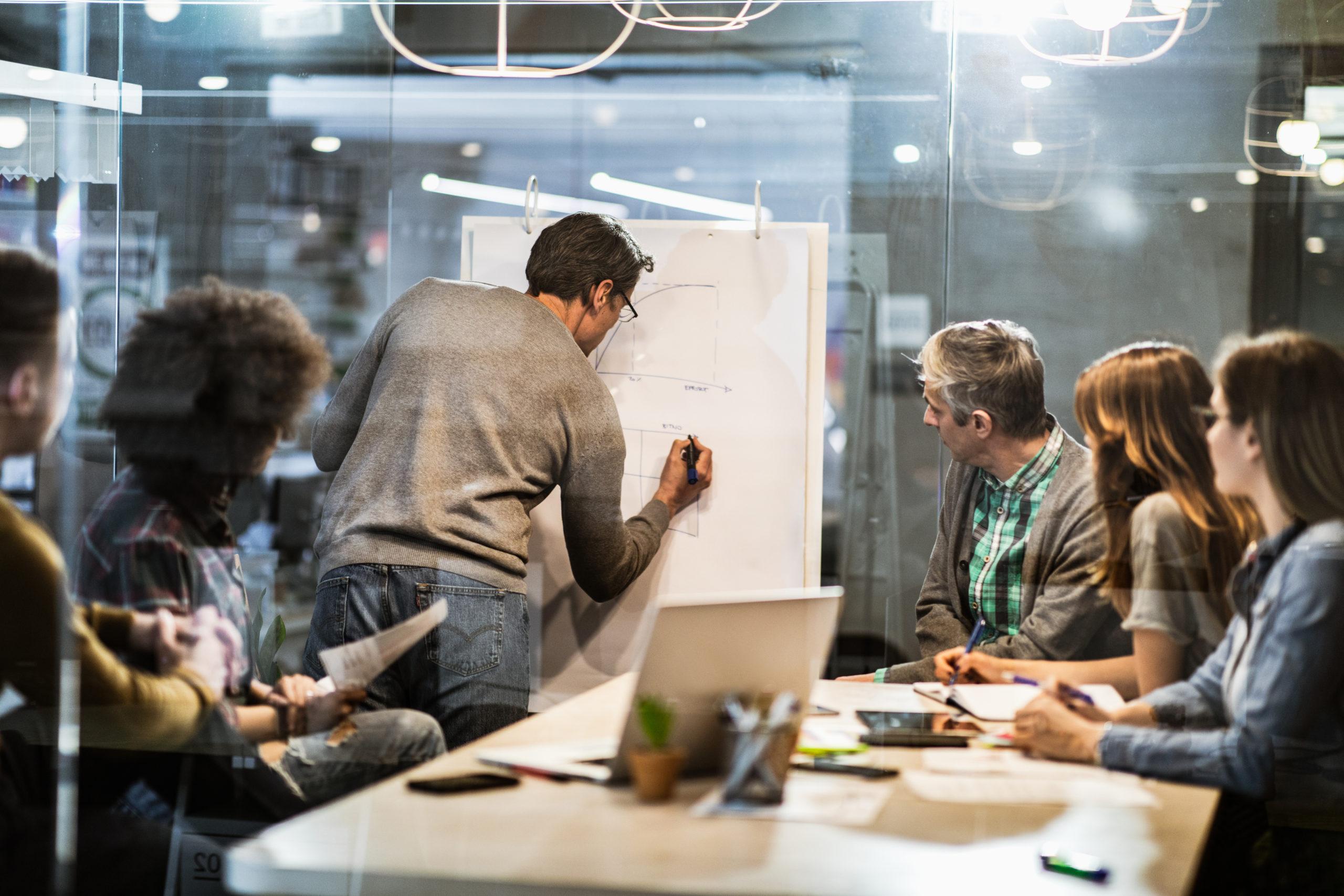
After you do a study or research, you will probably want to know if the results you got mean anything. More specifically, you want to know if there are statistically significant differences between the groups you studied. After all, statistically significant results help you know that what you studied—the variables you chose—are having an impact on the results.
For example, let’s say you did research on your customer base, and you wanted to determine if certain age groups bought your product more than others. Once you have your data, you need to determine if there’s any statistically significant difference between the age groups. How do you do that?
One way to determine if there are statistical differences between groups is to do an analysis of variance also called ANOVA. What is analysis of variance? Read on to learn more about ANOVA tests and how to use them for your own analyses.
What Is Analysis of Variance?
Analysis of variance or ANOVA is a statistical test developed by Ronald Fisher in 1918, and it’s been used by statisticians and researchers ever since. The analysis of variance test is a way to compare means from different groups and determine if the differences in those means are statistically significant. If they’re statistically significant, that means the variable for that group is having an impact on what you’re researching. If they’re not statistically significant, then your variables aren’t affecting what you’re studying.
Let’s revisit the example from earlier. You wanted to determine whether 18–24 years old, 25–35 years old, or 36–45 years old buy your products more. You gather all of your data about how much people are buying based on a random sampling. You determine the mean for each age group on product purchases. ANOVA is then how you can determine if there’s a statistically significant difference between those means.
An analysis of variance test will take into account the sample size and differences between means to give you an F value. The F value can then be analyzed to give you a probability or P value—or the probability that there’s a statistically significant difference. Let’s say you get a P value of 0.03. That would mean your results are statistically significant, and you can reject the null hypothesis. Most likely, that would mean you can determine that age is a significant variable in who buys your product, and you could consider making marketing decisions based on that. That’s the power of ANOVA.
How Does ANOVA Help?
At the core of it all, ANOVA helps you determine what variables have statistical differences and what variables are important to look at in more depth. Even more importantly, ANOVA can give you a glimpse into the motivation behind behavior. What’s driving customers to click on a link? ANOVA might help you determine that. Essentially, ANOVA helps by giving meaning to numbers, direction to actions.
Types of ANOVA
ANOVA is a broad category for several types of tests. The big two to discuss are one-way and two-way ANOVA tests. A one-way ANOVA test is the simplest form of ANOVA. For this test, you’ll need one independent variable and two or more levels. For example, you could use the months of the year as levels but still only test one variable. Two-way ANOVA or full factorial ANOVA is when you have two or more independent variables to test. Two-way ANOVA measures independent variables against each other and if independent variables affect each other.
There are a couple of other types of ANOVA to consider:
- Welch’s F Test ANOVA. The Welch’s F Test doesn’t assume the variances between groups are equal, which can be beneficial for some data sets. This type of unranked ANOVA test works when there are two assumptions that are true about the data:
- The sample size is 10 times greater than the calculation group (satisfying the Central Limit Theorem)
- There are few or not outliers in the data distribution
- Ranked ANOVA. If these assumptions above don’t hold, you can instead use a ranked ANOVA test. Ranked ANOVA tests can hold up against outliers and non-normal distributions because the values are replaced with a rank ordering.
- Games-Howell Pairwise Test. This ANOVA test doesn’t work with the assumption that variations between distributions are equal, and it’s a test when there’s a higher likelihood of finding statistically significant results.
ANOVA Terms to Know
There are some important terms to be familiar with to work with an analysis of variance test. Here’s ANOVA terminology that may be important for you:
- Independent variable. This is the variable that you choose to change, and you’re studying how it will affect the dependent variable.
- Dependent variable. Variables that don’t change and are instead affected by the independent variable.
- The null hypothesis. When you do an analysis of variance, you will have a pair of hypotheses. The null hypothesis will be the one that says there is no difference between the groups you’re looking at. If your p value is less than 0.05, you can reject the null. If not, you fail to reject the null hypothesis.
- The alternative hypothesis. Your other hypothesis is that there is a difference between the groups.
The Formula for Analysis of Variance
The formula for ANOVA is F = MST/MSE. That may look simple, but it involves a few more numbers. The MST is your total sum of squares (all of your means put through a formula) divided by the population total minus one. That gives you the top value for the ANOVA formula. MSE is your sum of squares with error divided by the number of observations minus one. Once you have both variables, you divide the MST by the MSE, and that gives you the F value. With your F value, you can use an ANOVA chart to determine the p value, which will tell you if you can reject the null hypothesis or if you fail to reject the null hypothesis.
How to Run an ANOVA
That formula can get tricky when a lot of numbers are involved, so not every statistician, researcher, or analyst does ANOVA by hand—though it can be calculated by hand, thanks to creation before computer programs. Instead, most researchers use computer software and programming to perform the test. R, Stata, SPSS, and Minitab are great choices for running an ANOVA test accurately and quickly.
Drawbacks to an Analysis of Variance Test
An ANOVA test will tell you that there is a difference between means and if that’s statistically significant. But ANOVA won’t tell you where that difference lies. For example, let’s return to our earlier example. You’re testing three age groups for your product purchases. The ANOVA test will tell you that age is statistically significant, but it won’t tell you which group is the one with the biggest difference. You’ll need to do additional statistical tests to determine which age group is most interested in your product.
Analysis Is Easy with Pearl-Plaza
Statistical tests can be overwhelming, including ANOVA. While it’s always possible to do an analysis of variance on your own, it’s usually easier and more accurate with some support. Pearl-Plaza is here to help. Whether you’re conducting a survey, research, or analysis of variance, the process just got a lot easier with Pearl-Plaza. Book your demo today and see how you can simplify your processes.