Addressing AI Hallucinations for Improved Business Performance
AI hallucinations occur when AI models generate incorrect or made-up responses. These hallucinations create challenges across industries relying on AI, causing customer dissatisfaction and reputational harm. Addressing this issue is key to improving business performance and customer experiences.
Think about the last time you asked ChatGPT a fairly simple question but got an unexpected response. Perhaps it provided a factually incorrect statement or just misunderstood your prompt. The result is described as a “hallucination”, a growing concern for businesses using AI systems.
What is an AI hallucination?
An AI hallucination occurs when an AI system produces false or misleading results as facts. A popular example is a large language model (LLM) giving a fabricated answer to a prompt it fails to understand.
Humans hallucinate when they see something that isn’t there. While AI models don’t “see” anything, the concept works well to describe their output when it’s inconsistent with reality. These hallucinations are mainly the result of issues with the training data. If the model is trained on insufficient or biased data, it’s likely to generate incorrect outputs.
An AI system is only as good as the data you feed it. It doesn’t “know” anything beyond its training data and has no concept of fact or fiction. An AI model like ChatGPT has one goal: predict the most appropriate response to a prompt. The problem is that its prediction can sometimes be well off the mark!
Types of AI hallucinations
There are various types of hallucinations, based on what a model contradicts:
- Prompt contradiction is when an LLM’s output is inconsistent with the information requested in the prompt. An example would be responding with an anniversary message to a prompt asking for a birthday card.
- Factual contradiction is when an LLM produces an incorrect answer as fact. For example, responding with “New York” to a question about the French capital.
- Random hallucination occurs when the model’s output has no connection with the prompt. If you ask for a chocolate cake recipe and receive a phrase like “Owls are nocturnal birds” in response, that would be a random hallucination.
- Sentence contradiction is when an LLM generates a sentence that contradicts its previous sentence. An example would be saying “Roses are red” only to say “Roses are purple” later in the output.
AI Hallucination Examples
- Stating obvious errors or false information as fact.
- Making up information and references.
- Misunderstanding the prompt.
- Providing incomplete information or context.
Generative AI has made impressive progress in content generation. However, it’s still capable of generating incorrect or misleading information. These hallucinations are a concern for AI in customer experience, affecting individuals and businesses alike. Here are some common examples of AI hallucinations in real-world systems.
Stating obvious errors or false information as fact
AI models sometimes generate text that is inconsistent with factual information. A famous example of this hallucination is Gemini’s incorrect response in a promotional video. The chatbot, formerly Bard, was asked, “What new discoveries from the James Webb Space Telescope can I tell my 9-year-old about?”
Gemini claimed that the JWST took the first image of a planet outside our solar system. This information is false since it was the European Southern Observatory’s Very Large Telescope (VLT) that took the first photos of an exoplanet back in 2004!
Making up information and references
AI models may invent details or references that don’t exist. For example, Google’s AI Overview generated this response to a prompt asking how long one should stare at the sun for best health:
“According to WebMD, scientists say that staring at the sun for 5-15 minutes, or up to 30 minutes if you have darker skin, is generally safe and provides the most health benefits.”
AI Overview states incorrect information here and wrongly attributes it to WebMD.
Similarly, speech-to-text AI tools that transcribe audio recordings are prone to hallucinations. For example, transcription tools tend to insert random phrases from their training data when they encounter a pause in the audio.
A concerning fact is that these phrases can be inaccurate and misleading, or even worse offensive and potentially harmful such as incorrect treatments in the case of medical transcriptions. Therefore, the inability of traditional AI tools to handle breaks in audio can have negative consequences for organizations.
Misunderstanding the prompt
A generative AI system may respond appropriately but still misunderstand your prompt. An example of this hallucination is asking ChatGPT to solve a Wordle puzzle.
While the system generates a coherent response, its solutions tend to be well off the mark. For instance, it may suggest a word that doesn’t match the pattern of letters you provide as input.
Providing incomplete information or context
Sometimes, AI models fail to respond comprehensively, leading to dangerous results. Once again, Google’s AI Overview provides an example of this occurrence. It generated largely correct information when asked which wild mushrooms are safe to eat.
However, it failed to specify how to identify fatal mushrooms. It suggested that mushrooms with “solid white flesh” are safe to eat, but it didn’t mention that some dangerous variants have the same feature.
What Problems Does AI Hallucination Cause?
AI hallucinations create challenges across various industries. Its inaccurate predictions and information hurt the customer experience, impacting the business’s reputation. Here are some of the problems these hallucinations cause in key sectors:
Healthcare
AI has become a significant part of healthcare workflows. Its ability to summarize patient information and even help with diagnoses is impactful. One of its most notable applications is transcribing medical visits. AI-powered transcriptions help doctors record and review patient interactions to make informed decisions.
It is vital to maintain accuracy and completeness in these transcriptions. A hallucination in the text would make it difficult to provide effective treatment and diagnoses.
For example, OpenAI’s Whisper, an AI-powered transcription tool, raised concerns by inventing phrases during moments of silence in medical conversations. Researchers found that Whisper was hallucinating in 1.4% of its transcriptions. This is a significant figure given that the tool had been used to transcribe around 7 million patient visits.
Some hallucinations were in the form of irrelevant text like “Thank you for watching!” during a conversation break in the transcription. Other instances were far more concerning, including fake medication like “hyperactivated antibiotics” and racial remarks. These hallucinations can have harmful consequences as they misinterpret the patient’s intent, leading to misdiagnoses and irrelevant treatments.
Contact Centers
In customer service, contact center AI hallucinations can damage brand credibility. Customers won’t be able to trust a business after getting an inappropriate response to their queries.
For example, a chatbot might give incorrect information about a product, policy, or support steps. Similarly, transcription tools often hallucinate phrases during pauses in agent-customer conversations. These hallucinations can provide an inaccurate view of the customer’s experience, resulting in poor analysis that fails to solve actual pain points.
Therefore, your CX program will suffer if it’s relying on inaccurate call center transcriptions. Despite your best intentions, a hallucination could be enough to cause customer dissatisfaction.
Unlike traditional tools, Pearl-Plaza’s advanced AI-powered solution addresses this specific problem to ensure your CX team accurately records customer interactions. As a result, you can be ensured you’re taking the right steps towards improving the customer experience.
Legal
AI enables legal professionals to save time on research and brief generation. Generative AI models can help produce drafts and summarize key points. However, due to hallucinations, relying on these models for crucial information like legal references can be tricky.
A law firm was fined $5,000 after its lawyers submitted fake citations hallucinated by ChatGPT in a court filing. The model invented six cases, which the lawyers used to support their arguments without verifying their accuracy. These cases were either not real, misidentified judges, or featured non-existent airlines.
Finance
In the financial sector, where precision is crucial, AI hallucinations can be costly. While AI systems can help crunch numbers, they can also hurt financial services reputation management efforts. Inaccurate financial reporting can affect investment decisions and stakeholder trust.
A popular instance is Microsoft’s first public demo of Bing AI. The model wrongly summarized a Q3 financial report for Gap, incorrectly reporting the gross and operating margins.
For example, the report stated a gross margin of 37.4 percent and an adjusted gross margin of 38.7% (excluding an impairment charge). However, Bing incorrectly reported the 37.4% margin as inclusive of adjustments and impairments.
Media and Journalism
Journalism suffers from AI hallucinations, such as fabricated quotes and inaccurate facts. While generative AI can help draft news stories and articles, it should combine human editing and verification to ensure accuracy. Otherwise, a single misstep like a misattributed quote can cause public backlash and reputational harm.
Education
The education sector has benefited from AI for research purposes. For instance, AI models are reasonably good at summarizing articles, generating ideas, and writing whole sections. Just like legal professionals, though, students and researchers must be extra careful with references.
For example, a librarian at the University of Southern California was asked to produce articles based on a list of 35 references provided by a professor. Despite her vast experience, the librarian couldn’t locate a single article. The professor eventually revealed that since ChatGPT invented these references, the articles simply didn’t exist!
This example highlights a common challenge for AI models. The National Institute of Health found that up to 47% of ChatGPT references are fabricated. Human oversight is essential to prevent incorrect citations and loss of trust.
Why Does AI Hallucinate?
- Low-Quality Training Data
- Overfitting
- Lack of Real-World Grounding
- Inability to Fact-Check
AI hallucinations are a by-product of how we design and train these systems. Common causes include:
Low-Quality Training Data
An AI model is only as good as the data you provide. Biased, outdated, and insufficient datasets will cause AI to generate inappropriate results. Even if it doesn’t understand your prompt, AI will craft a response based on its data, resulting in factual contradictions.
Overfitting
Even with the best training data, AI models will suffer if they can’t generalize to new data. An excellent accuracy score in the training phase sounds good in theory. But, what if the model is simply memorizing inputs and outputs? It won’t be able to produce accurate predictions or information when presented with inputs it hasn’t seen before. It’s important to prevent overfitting the model to ensure reliability in real-world systems.
Lack of Real-World Grounding
Many AI models are trained without real-world situational grounding. Think about the examples in which AI invents legal and academic references. These fabrications occur because AI struggles to understand real-world facts and physical properties. As a result, it produces outputs that look coherent but are inconsistent with reality.
Inability to Fact-Check
AI systems aren’t designed to fact-check information. They can only rely on patterns in the training data, even if they are incorrect or outdated. The lack of real-world understanding and fact-checking highlights the importance of human oversight for verification.
How to Prevent AI Hallucinations?
- Create restraints to limit outcomes
- High-quality training data
- Use data templates
- Combine with human oversight
- Provide clear, specific prompts
Preventing AI hallucinations requires specific prompting and improvements in training. Effective approaches include:
Create restraints to limit outcomes
AI models are trained to respond to prompts, even with little to no relevant information. This is how issues like inappropriate responses regarding dangerous mushrooms arise.
Therefore, it’s important to set restraints limiting the possible outcomes AI can generate. This occurs during the training phase, where you can provide examples and formats that encourage the AI to respond in a certain way. This prevents extreme outcomes and reduces the likelihood of hallucinations.
High-quality training data
The training data sets the foundation for generative AI results. High-quality training data is specific, complete, and free of biases. Using relevant data for a specific use case will enable the AI to produce consistently helpful outputs.
Use data templates
A template is helpful because it guides the AI model toward complete and accurate outputs. For example, if your model skips the introduction section in its articles, a template can encourage it to produce better responses. Data templates ensure consistency and reduce the likelihood of incorrect outcomes.
Combine with human oversight
Human oversight is valuable for ensuring AI accuracy. The models’ inability to fact-check their sources and ground their outputs in the real world can make them unreliable.
Regularly monitoring and reviewing AI outputs helps humans adjust AI performance for consistency and reliability. Human review also ensures the AI remains up-to-date with current trends and information. This prevents misinformation and improves model performance over time.
Provide clear, specific prompts
Clear prompts guide the AI toward the correct response. Specific and relevant inputs reduce the likelihood of inaccurate outputs. Vague prompts can lead to misinterpretation, resulting in hallucinations. Specific and targeted prompts help AI understand the context and expectations, improving response quality and relevance.
Can AI hallucinations be fixed?
You can prevent hallucinations by improving the training process and investing in good generative AI solutions.
For example, Pearl-Plaza’s CX-trained LLMs are specifically designed to address customer queries. It leverages sentiment analysis to understand customer intent and generate meaningful responses. As a result, your CX teams save time and effort that they can invest in building deeper customer relationships.
Pearl-Plaza AI is particularly useful for preventing hallucinations in transcribed conversations. Traditional AI systems hallucinate when they encounter pauses in conversations. Since they aren’t trained to handle moments of silence, they respond with random phrases from their training data. Think about how Whisper would include statements like “Thank you for watching!” in its medical visit transcriptions!
Pearl-Plaza’s solution works around this issue by detecting and removing all pauses in the audio file. As a result, it avoids hallucinating and processes all the words exchanged in an interaction to provide a complete and accurate transcription. This is helpful for healthcare and contact centers, enabling them to understand their clients and respond correctly.
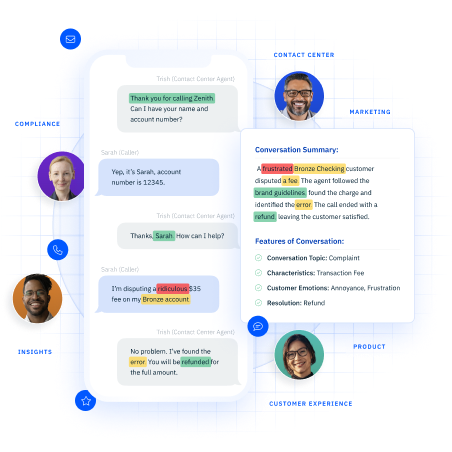
Will AI hallucinations go away?
According to experts like Meta’s Yann LeCun, AI hallucinations are unsolvable. However, advancements in training and prompt engineering will reduce these occurrences over time. Combining human oversight and good model design practices can help you address hallucinations before they impact your business.
Pearl-Plaza’s Awarding Advanced AI
AI hallucinations can impact business performance by providing inappropriate responses to customers. The good news is that the right generative AI solution can help prevent these hallucinations.
With the help of Pearl-Plaza Advanced AI, you can quickly generate complete and meaningful responses to customer feedback. It combines sentiment analysis, predictive modeling, and real-time insights to help you drive customer satisfaction and loyalty.