How to Use AI to Solve Real Business Problems
Instead of going for a high-risk moon-shot, here's how to effectively integrate AI into your business to solve tangible problems. In this article, originally posted on Forbes, Lexalytics CEO Jeff Catlin keeps it clear and concise.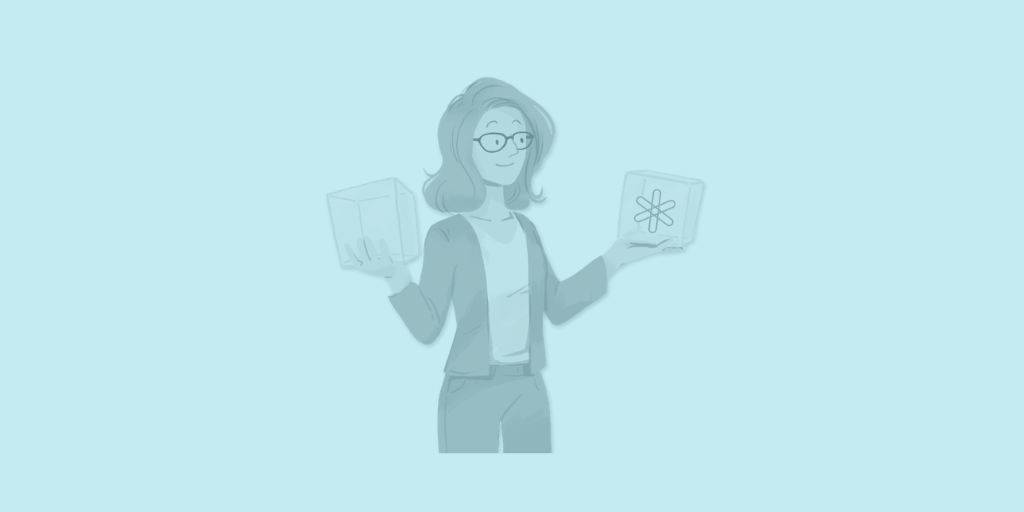
When people think AI, they often think big, such as curing cancer or solving climate change. Everybody is dreaming up the biggest problems possible and attempting to solve them with AI. Or there’s the flip side: not knowing what to do with AI and avoiding it accordingly. Hence why, according to McKinsey, just 20% of surveyed executives use AI-related technologies in their businesses.
There is a middle ground that will allow you to effectively integrate AI into your business without shooting for the moon (and blowing up on the launch pad). Look for business use cases where AI is already a proven solution — or an emergent one. And ensure that you have the data ecosystem available for AI to do its work.
With the right business case and the right data, AI can deliver powerful time and cost savings, as well as valuable insights you can use to improve your business.
Let’s take a look at a handful of business problems and how AI has been employed to solve them. These are practical, pragmatic, replicable efforts. It’s not intended to be a comprehensive list but instead a group of examples of “right-sized” projects.
The Problem: Predicting Customer Churn And Acting On It
VOZIQ provides customer experience management software to contact and call centers. (Full disclosure: VOZIQ and AlternativesPharma are Lexalytics, an pearl-plaza.rupany, customers.) For these centers, churn reduction is a major KPI. And they do so largely by using demographic and transaction history data.
However, this approach fails to capture the real-time, dynamic customer data picked up over the phone, much of which is recorded in notes taken by call center workers.
Rather than letting this data sit untapped, VOZIQ made use of it. It integrated AI to analyze post-call comments, categorizing them by topic and flagging sentiment scores that indicate customer dissatisfaction and the likelihood of churn. The company’s call center clients now receive insight into customer motivations, concerns and reasons for calling and are able to use this data to quickly spot and address customer churn.
The Problem: Creating Surveys That Deliver High-Quality Responses
SurveyMonkey is a leading survey software that lets businesses create and publish digital surveys in minutes. The system crunches an incredible 3 million responses every day. Since launching in 1999, SurveyMonkey has built up a powerful database of consumer and employee responses, and it’s now using AI to leverage this data.
It’s doing so in a few ways. One of them is by tapping into past survey results to help businesses create high-performing surveys with high completion rates. The system delivers real-time recommendations for adjusting which questions are asked and how in order to generate higher quality data. The data received by SurveyMonkey comes from unpaid survey-takers, so optimizing for high-quality responses is essential.
The company is also using AI to help organizations map customer feedback via sentiment analysis and to help vet candidates for jobs, scholarships and programs. Together these changes mark SurveyMonkey’s shift toward becoming a business intelligence tool.
The Problem: Reading And Handling Online Reviews
There are countless online reviews sites for guests, travelers and diners to post their experiences. But reading and reviewing them is no simple task. Reviews are scattered across a variety of sites, many of which use different formats. Add to this the challenge of unstructured, text-based reviews and the multilingual nature of the hospitality industry, and obtaining a comprehensive snapshot is a serious challenge.
But this is exactly the sort of situation where AI shines. For example, luxury hotel operator Dorchester Collection is using AI to monitor its own and competitor reviews to identify genuine guest needs. Using a platform called Metis, Dorchester Collection parses, summarizes and contextualizes reviews in order to gain insights, plan next steps and maintain a competitive advantage.
The Problem: Creating Messaging That Resonates With Users
What patients say in a clinical setting is different from what they say behind closed doors — or in the anonymity of the internet. AlternativesPharma is all too aware of this, which is why it uses qualitative data from web forums, social media and blogs in its efforts to help pharmaceutical marketing teams connect with both patients and doctors.
However, sourcing, collating and analyzing such data on a suitably large scale is impossible without the help of technology. To get the insights and in-depth analysis needed to improve pharmaceutical messaging and communications, AlternativesPharma turned to AI. This has allowed the company to analyze, categorize, and “theme” patients’ online discussions around particular diseases and pharmaceutical products. With new insights into how patients talk about certain ailments, AlternativesPharma has been able to help its clients more effectively communicate with patients and medical providers.
Building A Business Case For Your AI Problem
So how do you go about bridging the gap between AI as a possibility and AI as your chosen solution? Building a business case for AI isn’t so different from building one for any other business problem.
First, identify a need and a desired outcome (automation and efficiency are common drivers of successful AI projects). Then undertake a feasibility assessment. You’ll need to determine whether you have enough data to work with and whether it’s the kind of data that lends itself to pattern identification and subsequent decision making. You’ll need to make sure that the technology is sufficiently advanced to do what you need to do. If not, an existing solution may be the more cost-effective option.
Finally, you’ll need to ensure that the ROI of “success” is there. How will you measure your outcomes, and how will you incorporate these new understandings into your business model?
Implementing AI can be a big undertaking. But if you start with a business problem and take an incremental approach, you’ll be able to leverage its time and cost efficiencies to stay competitive both now and in the future.
This article originally appeared on Forbes Technology Council.
Further Reading: AI Use Cases, Ethics Concerns and More
AI in Education: Where is it Now and What is the Future?
How to Choose an AI Vendor – 4 Questions to Answer
Artificial Intelligence in Retail – 10 Present and Future Use Cases
AI in Healthcare: Data Ethics & Privacy Concerns
AI In Financial Services: Three Current And Emerging Applications